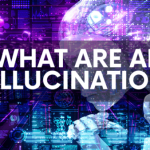
What Are AI Hallucinations?
March 13, 2024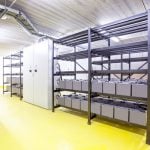
Data Center Battery Technology Explained
April 10, 2024The trouble with Big Data is that there is too much of it. An example is the data collected from video surveillance cameras for a small community. The community is safer because of the 100 or so video cameras covering the streets, parking lots, and intersections.
These cameras operate 24 hours per day, 365 days per year. They collect a total of 2,400 hours of video footage every day, which equals 876,000 hours of video footage each year. If human beings had to review this data for suspicious activity, at real-time speed, this would require a staff of 60 people. That is not economically feasible.
The only way to deal with this amount of collected information is to manage it with data-scanning and analytics using artificial intelligence software algorithms.
What Are the Key Offerings for Big Data Processing?
For companies that want to process Big Data, they need robust IT computer systems, AI programming, consultants for analytics, and support services. Here are some considerations:
- On-Premises Mainframes vs. Off-Site Servers: Major international conglomerates can afford the large mainframe systems to process Big Data on-premises. However, most companies choose dedicated servers or co-location servers, which provide the processing power needed for the analysis of Big Data. This IT solution is managed off-site. The advantages of having servers off-site include outsourcing the maintenance of them, lowering capital investment in facilities, and having the flexibility to increase IT services as needed.
- AI Programming: Data mining of Big Data is achieved by using AI programming that works with algorithms to find patterns in the Big Data that are noteworthy. This provides insights that help management make better-informed decisions.
- Consultants for Analytics: It is cost-effective for most companies to outsource the IT consultant work to develop their analytics of Big Data. These specialists can be engaged on an “as-needed” basis to construct an analytics program that is suitable for a company’s requirements.
- Support Services: Support services include managed hosting, IP transit services, and connections to the cloud.
How Can Big Data Be Managed Effectively?
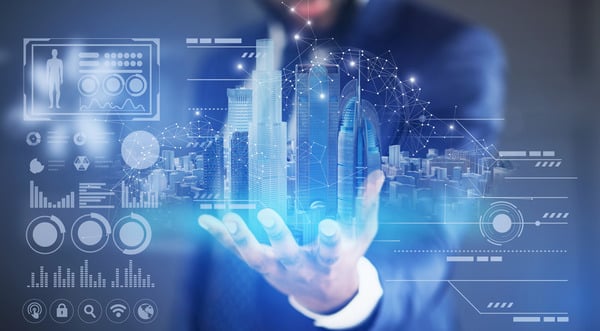
Big Data is managed well by using large data centers that are strategically placed. The main benefit of using redundant processing systems that are physically located in separate geographic areas is that any localized failure, such as one caused by a natural disaster, does not take the entire system down.
Business-critical hardware needs to have at least triple redundancy to achieve 99.9% uptime performance. Foundational IT structures consider the risk of calamities and plan for those that can impact an IT network. Load-balancing, in real-time, manages any partial systemic failure of some network servers to re-route the processing to the servers that remain operating in the network.
What Are the Best Practices for Big Data Storage Management?
Data storage requirements for Big Data are substantial. One approach is to capture and process the localized data and then forward the storage to a more extensive storage system that is maintained in the cloud.
Another approach is to use a “virtualized” data system that creates a virtual layer of the data. This virtual layer knows where the data is stored on the network. When calculations are being made using the AI algorithm in a virtual system, only the data needed for that specific calculation is accessed. The original data storage remains intact and in place, without the need for copying data files
This approach utilizes a network-wide data management protocol. It reduces the need for data storage memory as well as improves the computational processing speeds.
How Is Artificial Intelligence Transforming Data Analysis?
Firstmark produced an infographic chart that shows the 2019 Data & AI Landscape. The trend of moving Big Data computational processing to the cloud is undisputable. Forbes reports that distributed data storage is being rapidly replaced by storing Big Data on the cloud and then data mining using SaaS AI programs.
A major digital transformation allows companies to improve management decisions and discover insights that lead to innovation. The change comes from deep learning. Deep learning is a technique of using AI programming to enhance its functions through machine learning.
No human intervention or detailed programming by human beings, for each conceivable instance, is necessary. Instead, a set of algorithms are designed that the programming uses to learn by the application of the algorithms to a large data set.
How Are AI and Big Data Interconnected in Today’s Technology?
AI and Big Data are being used increasingly by companies of modest size. They access the IT hardware resources available from data centers. Then, they apply the AI tools available as cloud services to the Big Data that they collect.
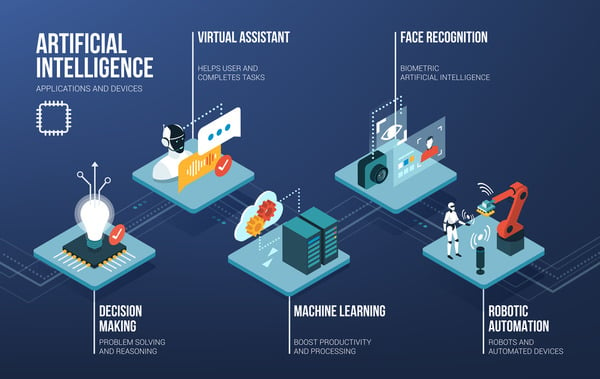
DZone notes that some ways that AI is applied to Big Data Analytics include:
- Detecting Anomalies: AI can analyze Big Data to detect anomalies (unusual occurrences) in the data set. This can be applied to networks of sensors and parameters that have a predefined appropriate range. Any node of the network that is outside of the range is identified as a potential problem that needs attention.
- Probabilities of Future Outcomes: AI can analyze Big Data using Bayes theorem. The likelihood of an event occurring can be determined using known conditions that have a certain probability of influencing the future outcome.
- Recognizing Patterns: AI can analyze Big Data to look for patterns that might otherwise remain undetected by human supervision.
- Data Bars and Graphs: AI can analyze Big Data to look for patterns in bars and graphs that are made from the underlying data set.
Another key driver of this trend is that Big Data is increasing through the explosion of connected devices being deployed with the expansion of the Internet of Things (IoT).
How Are IoT and AI Together Enhancing Big Data’s Future and Security?
The Internet of Things is a key driver of Big Data and AI’s evolution. With estimates predicting over 29 billion IoT devices by 2030, the large amounts of data generated are set to exponentially expand Big Data. This surge both increases the data for AI to analyze and also shows how crucial it is to use advanced analytics to get useful insights from the worldwide IoT network.
AI is useful for identifying people using biometric data such as facial recognition, fingerprints, and retinal scans of eyes. AI is key in improving security and trust in technology by using biometric data like facial recognition, fingerprints, and retinal scans to identify people accurately. This role of AI in managing and protecting data is essential for dealing with the challenges of a world full of data and connections, highlighting how IoT and AI can change the future of technology.
What Infrastructure Challenges Do AI and Big Data Face?
The growth of Generative AI (GenAI) and Big Data is driving technology forward, greatly affecting data analytics and machine learning. However, this advancement faces a crucial challenge: the scarcity of AI GPUs and the urgent need for advanced data center infrastructure.
Data center operators, and the tech industry as a whole, are encountering delays in securing essential components for infrastructure expansion, leading to increased costs and potential innovation bottlenecks. This issue potentially affects smaller operators who could find it challenging to find the necessary space and power for high-performance computing services.
The increasing need for data centers, driven by a predicted doubling of data by 2027, requires building energy-efficient facilities to meet the high-power needs of GenAI. Shifting to liquid cooling and other new cooling methods over old ones highlights the need for updated data center designs to support the growing demands of AI and Big Data processing.
How Can We Overcome Infrastructure Challenges for a Sustainable AI and Big Data Future?
Facing the quick changes in AI and Big Data, the lack of GPUs, and the urgent need for better data centers show how critical the situation is for technology today. These challenges also create chances to innovate and make our digital infrastructure strong and lasting.
Tech companies, infrastructure providers, and regulators will need to come together to embrace green technologies, renewable energy, and better cooling methods. This will help us keep up with the digital age. By doing this, we can fully use AI and Big Data to improve our future responsibly and sustainably.